Defining artificial intelligence (AI) is a complex task, often subject to evolving perspectives. Definitions based on goals or tasks can change as technology advances. For instance, chess-playing systems were a focus of early AI research until IBM's Deep Blue defeated grandmaster Gary Kasparov in 1997, shifting the perception of chess from requiring intelligence to brute-force techniques.
On the other hand, definitions of AI that tend to focus on procedural or structural grounds often get bogged down in fundamentally unresolvable philosophical questions about mind, emergence and consciousness. These definitions do not further our understanding of how to construct intelligent systems or help us describe systems we have already made.
The Turing Test - A Measure of Machine Intelligence

The Turing test, often seen as a test of machine intelligence, was Alan Turing's way of sidestepping the intelligence question. It highlighted the semantic vagueness of intelligence and focused on what machines can do rather than how we label them.
“The original question ‘Can machines think?’ I believe to be too meaningless to deserve discussion. Nevertheless, I believe that at the end of the century, the use of words and general educated opinion will have altered so much that one will be able to speak of machines thinking without expecting to be contradicted.” - Alan Turing
In the end, it is a matter of convention, not terribly different than debating whether we should refer to submarines as swimming or planes as flying. For Turing, what really mattered was the limits of what machines are capable of, not how we refer to those capabilities.
Measuring Human-Like Thinking in AI
To that end, if you want to know if machines can think like humans, your best hope is to measure how well the machine can fool other people into thinking that it thinks like humans. Following Turing and the definition provided by the organizers of the first workshop on AI in 1956, we similarly hold that “every aspect of learning or any other feature of intelligence can in principle be so precisely described that a machine can be made to simulate it.”
To achieve human-like performance or behavior in any given task, AI should be capable of simulating it with a remarkable level of precision. The renowned Turing test was designed to assess this ability by evaluating how effectively a computer or machine could deceive an observer through unstructured conversation. Turing's original test even called for the machine to convincingly portray a female identity.
Assessing AI's Human-Level Understanding
In recent years, significant advancements in machine learning techniques, coupled with the abundance of extensive training data, have enabled algorithms to engage in conversation with minimal understanding. Furthermore, seemingly insignificant tactics, such as deliberately incorporating random spelling mistakes and grammatical errors, contribute to algorithms becoming increasingly persuasive as virtual humans, despite lacking genuine intelligence.
Novel approaches to assessing human-level comprehension, such as the Winograd Schemas, propose querying a machine about its knowledge of the world, object uses, and affordances that are commonly understood by humans. For instance, if we were to ask the question, "Why didn't the trophy fit on the shelf? Because it was too big. What was too big?" any person would immediately ascertain that the trophy was the oversized element. Conversely, by making a simple substitution—"The trophy didn't fit on the shelf because it was too small. What was too small?"—we inquire about the inadequacy in size.
In this scenario, the answer unequivocally lies in the shelf. This test, with heightened precision, delves into the depths of machine knowledge pertaining to the world. Simple data mining alone cannot provide an answer. This definition necessitates that an AI has the capability to emulate any facet of human behavior, drawing a meaningful distinction from AI systems specifically designed to demonstrate intelligence for particular tasks.
Differentiating Types of AI and Their Learning Methods
Artificial General Intelligence (AGI)
Artificial General Intelligence (AGI), commonly known as General AI, is the concept most frequently discussed when referring to AI. It encompasses the systems that evoke futuristic notions of "robot overlords" ruling the world, capturing our collective imagination through literature and film.
Specific or Applied AI
Most of the research in the field focuses on specific or applied AI systems. These encompass a wide range of applications, from Google and Facebook's speech recognition and computer vision systems to the cybersecurity AI developed by our team at Vectra AI.
Applied systems typically leverage a diverse range of algorithms. Most algorithms are designed to learn and evolve over time, optimizing their performance as they gain access to new data. The ability to adapt and learn in response to new inputs defines the field of machine learning. However, it's important to note that not all AI systems require this capacity. Some AI systems can operate using algorithms that don't rely on learning, like Deep Blue's strategy for playing chess.
However, these occurrences are typically confined to well-defined environments and problem spaces. Indeed, expert systems, a pillar of classical AI (GOFAI), heavily rely on preprogrammed, rule-based knowledge instead of learning. It is believed that AGI, along with the majority of commonly applied AI tasks, necessitate some form of machine learning.

The Role of Machine Learning
The figure above shows the relationship between AI, machine learning, and deep learning. Deep learning is a specific form of machine learning, and while machine learning is assumed to be necessary for most advanced AI tasks, it is not on its own a necessary or defining feature of AI.
Machine learning is necessary to mimic the fundamental facets of human intelligence, rather than the intricacies. Take, for instance, the Logic Theorist AI program developed by Allen Newell and Herbert Simon in 1955. It accomplished the proof of 38 out of the initial 52 theorems in Principia Mathematica, all without any need for learning.
AI, Machine Learning, and Deep Learning: What's the Difference?
Artificial intelligence (AI), machine learning (ML), and deep learning (DL) are often mistaken for being the same thing, but they each have their own unique meanings. By understanding the scope of these terms, we can gain insight into the tools that leverage AI.
Artificial Intelligence (AI)
AI is a broad term that encompasses systems capable of automating reasoning and approximating the human mind. This includes sub-disciplines like ML, RL, and DL. AI can refer to systems that follow explicitly programmed rules as well as those that autonomously gain understanding from data. The latter form, which learns from data, is the foundation for technologies like self-driving cars and virtual assistants.
Machine Learning (ML)
ML is a sub-discipline of AI where system actions are learned from data rather than being explicitly dictated by humans. These systems can process massive amounts of data to learn how to represent and respond to new instances of data optimally.
Video: Machine Learning Fundamentals for Cybersecurity Professionals
Representation Learning (RL)
RL, often overlooked, is crucial to many AI technologies in use today. It involves learning abstract representations from data. For example, transforming images into consistent-length lists of numbers that capture the essence of the original images. This abstraction enables downstream systems to better process new types of data.
Deep Learning (DL)
DL builds on ML and RL by discovering hierarchies of abstractions that represent inputs in a more complex manner. Inspired by the human brain, DL models use layers of neurons with adaptable synaptic weights. Deeper layers in the network learn new abstract representations, which simplify tasks like image categorization and text translation. It's important to note that while DL is effective for solving certain complex problems, it is not a one-size-fits-all solution for automating intelligence.

Reference: “Deep Learning,” Goodfellow, Bengio & Courville (2016)
Learning Techniques in AI
Far more difficult is the task of creating programs that recognize speech or find objects in images, despite being solved by humans with relative ease. This difficulty stems from the fact that although it is intuitively simple for humans, we cannot describe a simple set of rules that would pick out phonemes, letters and words from acoustical data. It’s the same reason why we can’t easily define the set of pixel features that distinguish one face from another.

The figure on the right, taken from Oliver Selfridge’s 1955 article, Pattern Recognition and Modern Computers—shows the same inputs and can lead to different outputs, depending on the context. Below, the H in THE and the A in CAT are identical pixel sets but their interpretation as an H or an A relies on the surrounding letters rather than the letters themselves.
For this reason, there has been more success when machines are allowed to learn how to solve problems rather than attempting to predefine what a solution looks like.
ML algorithms have the power to sort data into different categories. The two main types of learning, supervised and unsupervised, play a big role in this capability.
Supervised Learning
Supervised learning teaches a model with labeled data, enabling it to predict labels for new data. For example, a model exposed to cat and dog images can classify new images. Despite needing labeled training data, it effectively labels new data points.

Unsupervised Learning
On the other hand, unsupervised learning works with unlabeled data. These models learn patterns within the data and can determine where new data fit into those patterns. Unsupervised learning doesn't require prior training and is great at identifying anomalies but struggles to assign labels to them.

Both approaches offer a range of learning algorithms, constantly expanding as researchers develop new ones. Algorithms can also be combined to create more complex systems. Knowing which algorithm to use for a specific problem is a challenge for data scientists. Is there one superior algorithm that can solve any problem?

The 'No Free Lunch Theorem': No Universal Algorithm Exists
The "No Free Lunch Theorem" states that there is no one perfect algorithm that will outperform all others for every problem. Instead, each problem requires a specialized algorithm that is tailored to its specific needs. This is why there are so many different algorithms available. For example, a supervised neural network is ideal for certain problems, while unsupervised hierarchical clustering works best for others. It's important to choose the right algorithm for the task at hand, as each one is designed to optimize performance based on the problem and data being used.
For instance, the algorithm used for image recognition in self-driving cars cannot be used to translate between languages. Each algorithm serves a specific purpose and is optimized for the problem it was created to solve and the data it operates on.
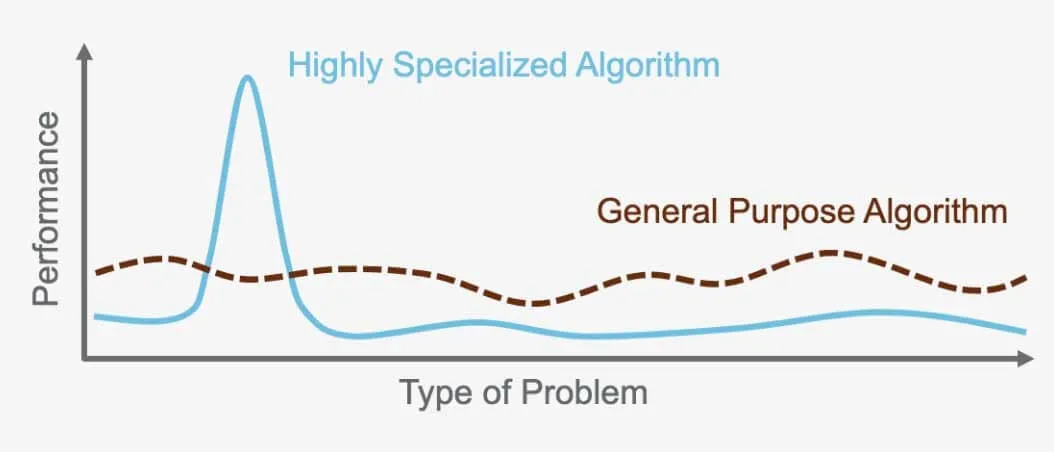
Selecting the Right Algorithm in Data Science
Choosing the right algorithm as a data scientist is a blend of art and science. By considering the problem statement and thoroughly understanding the data, the data scientist can be guided in the right direction. It's crucial to recognize that making the wrong choice can lead to not just suboptimal results, but completely inaccurate ones. Take a look at the example below:
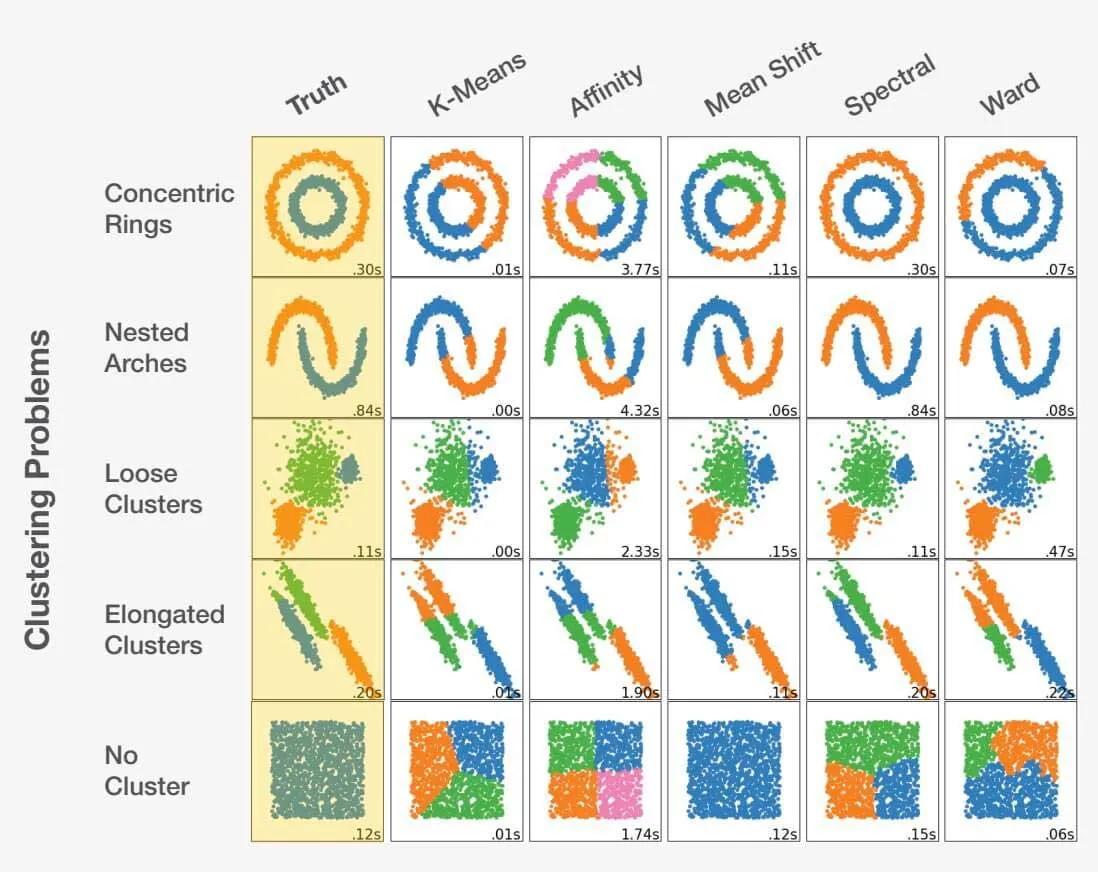

Adapted from scikit-learn.org.
Choosing the right algorithm for a dataset can significantly impact the results obtained. Each problem has an optimal algorithm choice, but more importantly, certain choices can lead to unfavorable outcomes. This highlights the critical importance of selecting the appropriate approach for each specific problem.
How to Measure an Algorithm's Success?
Choosing the right model as a data scientist involves more than just accuracy. While accuracy is important, it can sometimes hide the true performance of a model.

Let's consider a classification problem with two labels, A and B. If label A is much more likely to occur than label B, a model can achieve high accuracy by always choosing label A. However, this means it will never correctly identify anything as label B. So accuracy alone is not enough if we want to find B cases. Fortunately, data scientists have other metrics to help optimize and measure a model's effectiveness.
One such metric is precision, which measures how correct a model is at guessing a particular label relative to the total number of guesses. Data scientists aiming for high precision will build models that avoid generating false alarms.

But precision only tells us part of the story. It doesn't reveal whether the model fails to identify cases that are important to us. This is where recall comes in. Recall measures how often a model correctly finds a particular label relative to all instances of that label. Data scientists aiming for high recall will build models that won't miss instances that are important.

By tracking and balancing both precision and recall, data scientists can effectively measure and optimize their models for success.