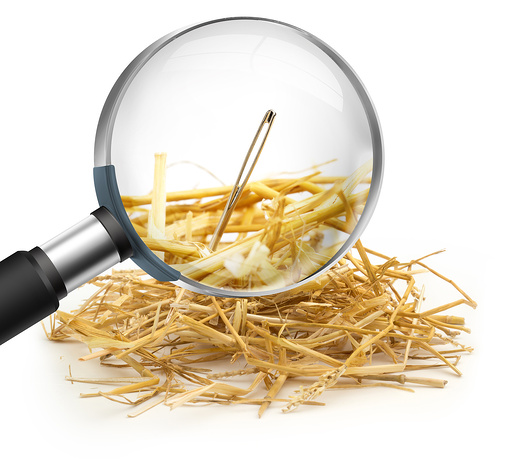
In the previous posts, we have examined the insider threat from various angles and we have seen that insider threat prevention involves the information security, legal and human resources (HR) departments of an organization. In this post, we want to examine what information security departments can actually do to detect ongoing insider threats, and even prevent them before they happen.
The literal needle in the haystack
Overall, insider threats represent only a small proportion of employee behavior. And while only the ‘black swan’ incidents become public knowledge, minor incidents such as theft of IP or customer contact lists will add up to major costs for organizations.
In addition, insiders are by default authorized to be inside the network and are both granted access to and make use of key resources of an organization. Given the large pile of access patterns visible in an organization’s network, how is one to know which ones are negligent, harmful or malicious behavior?
IT departments typically respond to the insider threat, if at all, by extensive monitoring and logging. The aim is to at least be able to do forensic analysis when a threat is happening and doing damage, and support the legal department with any investigations.
Obviously, such an approach will not help prevent the threat in any way. Recent updates to monitoring solutions such as Sure View and research programs of the US government have started taking a more proactive approach to detect a threat while it's happening, and even before it happens. We have seen that the psychology of the insider is very complex and that the insider typically takes precautions to evade detection, so how could a software solution reliably identify what is a threat and what is not?
Data science … the new solution to the insider threat problem?
The problem of detecting the insider threat before it actually happens is as difficult and complex to solve as the prediction of human behavior itself. What is the next action of a person? Which action will be inside the scope of assigned work for that person? Which action will indicate the preparation for an attack by that person?
Recent technological advances have shown significant improvements in predicting what was previously considered unpredictable – human behavior. Despite some initial setbacks, systems such as Google Now, Siri, or Cortana aim to predict users’ needs before they even know them.
This is becoming possible due to the vast amounts of behavioral data that has been collected and indexed, and the computational resources available for analysis have reached a critical mass for the deployment of large-scale artificial intelligence methods such as voice recognition, image analysis and machine learning. The term for this new predictive analysis of large amounts of behavior data is data science.
It is nowadays applied to various problems and areas, and could similarly be applied to the insider threat problem. As described above, an insider’s behavior is per definition authorized inside an organization’s network and there is typically not enough information available to derive an insider’s intention or psychology in real-time. However, as the amount of collected behavior data increases, there are more and more cues that could be revealed.
An initial data science approach is to learn commonly known indicators for insider threat behavior. These might be authorized behaviors, but are typically associated with a an insider who has veered off course. An example is exfiltration behaviors such as uploading data to a dropbox account, extensive use of USB sticks or high volume of downloads from internal servers. These indicators are specific enough to catch an ongoing attack, but only a limited set of attack types can be detected in this way (those for which the indicators are known).
In order to catch future -- and unknown -- attacks, a second approach is to focus on anomalies in observed behavior. An anomaly is something that deviates from what is standard, normal, or expected.
In the realm of behavior, a data science solution will analyze behavioral data and learn what is normal. ‘Normal’ behavior can refer to normalcy with regard to all observed behavior variations, an individual’s behavior over time or even social behaviors. Once a baseline of normalcy is established, outliers can be identified.
Knowing that insider threats are paired with changes in behavior of the individual in question, anomaly detection will reveal these, even in the early stages of a threat. However, this improved detection comes with a price: a higher number of false positives. Benign changes in behavior (e.g., due to job function or team changes, or coming back to work after the holidays etc.) will trigger detections and the amount of these detections can become overwhelming.
A third (and most advanced) data science approach is to generate narratives from output of the first and second approaches, i.e. combine indicators and anomalies to generate an understandable interpretation of the behavior going on inside an organization. The latter is obviously a hard nut to crack because, ultimately, it will involve creating a truly artificial intelligence. But we are getting there…
This article was originally published as part of the IDG Contributor Network.