Imagine having a security tool that thinks the way you teach it to think, that takes action when and how you have trained it to act. No more adapting your work habits to generic rules written by a third party and wondering how to fill in security gaps that the rules did not tell you about.
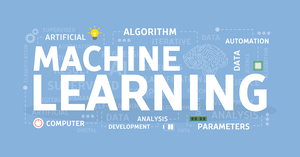
Machine learning, the cornerstone of Network Traffic Analytics (NTA), is the technology that acts on your behalf to increase your visibility into the infrastructure, enhance the detection of active threats, and simplify recovery from the threats that really matter.
We have become so indoctrinated to the deterministic orientation of rules that we frequently overlook its limitations. Think of rules as a cascade of IF…THEN…ELSE statements that an analyst creates to follow a known path. Any changes to the rules, say from including new operational data or from codifying new threat detection logic, brings undesired administrative burdens.
Even worse, the disconnects between hard-coded rules, evolving IT processes and ever-creative new threats saddle security operations to deal with heightened levels of false positives and inaccurate security profiles. With rules firing off alerts left, right and center, it’s crazy to think security operations can find and resolve the most critical problems.
Machine learning—both supervised and unsupervised—empowers human analysts by automating the manual and mundane parts of their jobs, such as threat detection, triage, correlation and scoring. However, it cannot be effective without a human working with it. Human analysts apply contextual knowledge and critical thinking to know whether a detection is a true problem because of their insights. Your job would be so much easier if only the security tools would listen to you!
Data scientists who build machine learning algorithms start with the results of human analysts who determine “this is a threat, this is okay, this bears further study.” The data scientist then works backwards from your given results to discover relationships in the data, so an algorithm can automate your threat-hunting approach to collecting data, detecting threats, and remediating the problem.
Machine learning automates what you, the security analyst, has taught it, allowing you to move on to solving other problems. You are always in control, correcting and guiding the machine learning tool to make you more effective in your job.
NTA applies machine learning to network data to give you advanced visibility, detection, and remediation powers. It helps you see exactly what is happening in your environment, which detections require immediate attention, and what remediation steps are most acceptable to users. It’s not magic; you are teaching the tool how it looks at security every step of the way. For example, static rules might trigger alerts when they see increased beaconing and DNS activity followed by large data exchanges to new servers in a new cloud domain. You know these alerts are false positives because your company is deploying new application workloads to a secondary cloud provider.
Or maybe you know it is an attack—but this is something only you can know. Do you want to spend time rewriting and debugging rules or would you prefer to simply tell your NTA tool to “learn this now?” Machine learning gives you the opportunity to continuously monitor your operating environment. It puts technology to work in bringing suspicious actions to your attention without the overhead of administering rules.
For instance, you do not want to burden privileged users with rigid rules that impede their ability to do their jobs. However, you can ask NTA to learn which privileged users access which servers, when, from where, and which protocols are used. Furthermore, you can guide your security tool on how to act when deviations are detected. Maybe you want the user to confirm that they wish to proceed, maybe you want granular activity-logging enabled, maybe the new protocol is unsecure and should be blocked for administrative actions.
Machine learning collects operational data in your environment to help you understand when problems arise and helps you make quick decisions about what to do about it. And it does this without requiring you to continually correct rules or define rules so broadly that they provide only false security. Some fear that machine learning, artificial intelligence and deep learning are destined to replace human security analysts. Our experience shows that machine learning has only empowered security analysts.
They have increased human security analyst productivity and job satisfaction—in much the same way that our lives have improved with apps that learn what we like to search for, send messages to, and how we like to travel. We observe similar trends following us in our professional lives. As a security analyst, you’ll discover a world of new possibilities as machine learning gives you a unique opportunity to showcase your creativity, decision-making and ability to get the most out of technology.