Today, the amount of encrypted web traffic is up 95% from over a decade ago, with much of it relying on TLS encryption for secure client-server communication. Services like Let's Encrypt have made it more accessible and cost-effective to implement HTTPS, ensuring our online privacy. However, this encryption also poses challenges, particularly for security professionals working to detect encrypted command-and-control (C2) channels used by cyber attackers. In this blog post, we'll explore the importance of C2 detection, the role of machine learning in security and how Vectra AI is leading the way in enhancing online security.
The attacker perspective
As an attacker, there is a drive to remain hidden in today’s hybrid environments. Wherever an attacker lands, a command and control (C2) channel must be established to blend in and hide their communications. The MITRE ATT&CK framework outlines many of the common types of C2 channels, such as multistage channels, multi-hop proxies, and HTTPS tunnelling. These channels are often encrypted using TLS or by masquerading as HTTPS traffic to blend in with web traffic (which is mostly HTTPS) and to encrypt communications and make detection more difficult.
Attackers also make use of further obfuscation techniques which make detecting C&C channels even harder. Not only is there the use of encryption inside communications channels to thwart analysis, but techniques such as jitter (creating more random timings for beacons to defeat looking for regular beacons) and padding.
There is sometimes even a further layer of encryption inside the communications channels to stop defenders from reading what data is being sent, and time-based variables such as session jitter to mask regular beaconing behaviour over time.
The security team perspective
Finding C&C channels in modern internet traffic is paramount for security analysts. The task is hampered not only by the encryption of attacker traffic, but also because much of the internet now functions on encrypted traffic and uses WebSockets and long open sessions which can look like C&C behaviour. To this end, analysts need to look at not just the raw traffic, but the metadata of that traffic. Flow traffic can be useful, but examining the deeper metrics about these flows such as time intervals of transmission, can expose malicious traffic in encrypted sessions.
Fortunately, Vectra AI's flow engine offers this depth of analysis for each tracked data flow, with sampling as granular as half-second intervals. This includes metrics such as bytes sent and received over time, providing insight into the dynamics of each interaction.
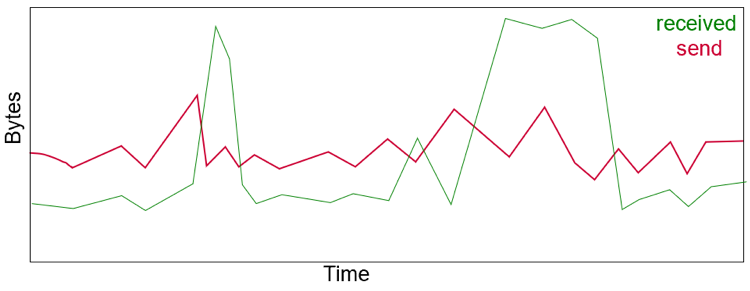
Machine Learning for C&C Detection
Vectra AI's journey in effectively utilizing time-domain data to detect command-and-control channels has seen various approaches, with a strong focus on supervised machine learning techniques. Several machine learning algorithms working in tandem offer both a coarse and granular view of attacker behaviors:
- Random Forests: Comprising multiple decision trees, random forests excel at delivering coarse-grained telemetry. They monitor time-series windows and track over 20 features, including client/server data ratios, data consistency, server break frequencies and session length.
- Recurrent Neural Networks (RNNs): RNNs enable the representation of temporal behaviors, where one sequence influences the next, revealing unique human-driven features. Essentially, RNNs mimic human memory.
- Long Short-Term Memory (LSTM) Deep Learning Neural Networks: LSTM networks can learn long-range temporal dependencies and relationships, including the ability to forget. This approach is versatile and applicable across various use cases, including natural language processing. In security, it allows for tracking relevant attacker activity patterns over extended periods.
The Power of Convergence
The convergence of these machine learning methods, each meticulously developed and fine-tuned over the years, empowers Vectra AI to comprehend the behaviors of encrypted traffic and generate high-fidelity alerts for command-and-control detection. Success hinges on the careful application of these methods and the quality of training data used.
Vectra AI is fortunate to have an award-winning team of data scientists and security researchers dedicated to algorithm development, sample curation and ongoing algorithm optimization for peak performance.
Conclusion
Detecting and prioritizing encrypted command-and-control channels with precision and minimal noise is a formidable challenge. It goes beyond the capabilities of a simple anomaly detector. Achieving this level of security requires the right data, the most suitable machine learning methods, and a dedicated team with expertise in building and fine tuning models. Vectra AI stands at the forefront of this mission, contributing to a safer online environment for all.