Measuring and improving total time of response is easier said than done. The reality is many organizations do not know their existing state of readiness to be able to respond to a cybersecurity incident in a fast, effective manner. And most don’t know what their level of risk awareness needs to be or an appropriate level of response. As mentioned in my previous blog, the classification of risk drives the necessary maturity level of the organization.
More critically, even when the risk is known, lack the personnel or staff inefficiencies will not result in an effective program. A big percentage of a security analyst’s time is spent addressing unexpected events that an existing process cannot handle. Security analysts perform a tremendous amount of tedious, manual work to triage alerts, correlate them and prioritize them. They often spend hours doing this only to learn that the alert is not actually a priority.
In addition, performing tedious, manual work introduces human errors. People excel at critical thinking and analysis, not repetitive manual work. Organizations have no recourse but to hire more people, reduce the workload or both. Achieving the desired response time for a high-level of threat awareness requires a thorough understanding of what tasks to automate and more importantly, when not to automate.
An efficient incident response process will keep people in the loop without giving them all the keys to the machines. Instead, the goal is to free-up the security analyst’s time to focus on higher-value work that requires critical thinking.
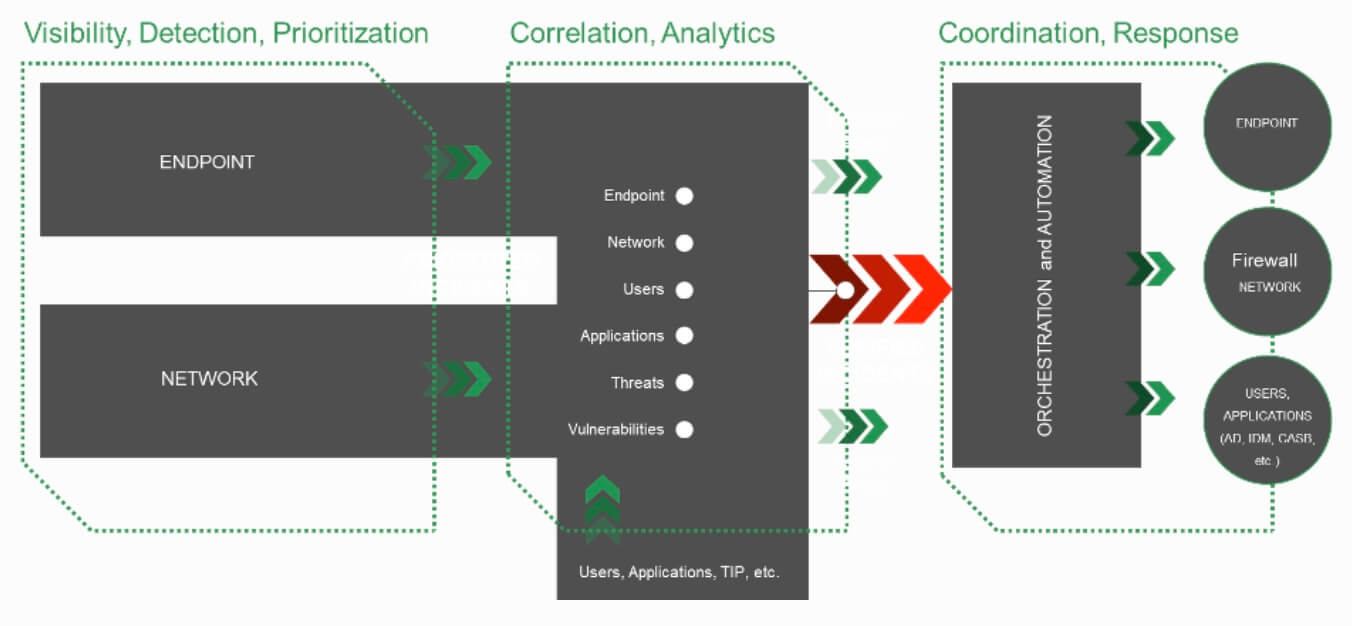
The model above has three stages that show how automation can be applied to a detection and response process. It breaks down this way:
- Visibility, detection and prioritization of attack indicators from endpoints and networks.
- Analysis of endpoint and network data correlated with other key data sources.
- A coordinated attack response across endpoints, networks, users, and applications.
Stage 1: Visibility, detection and prioritization
The network and its endpoints provide visibility and detection capabilities. They build upon visibility and detection data to provide the initial prioritization of an incident and immediate alerts. Automation of the detection and triage process at this stage reduces the total number of reported events by rolling up numerous alerts to create a single incident to investigate that describes a chain of related activities, rather than isolated alerts that a security analyst has to piece together. Assets and accounts central to an incident are contextualized and prioritized for threat and certainty. This information is then handed off to the next stage.
Stage 2: Correlation and analytics
In this stage, network and endpoint data are correlated with data from user, vulnerability and application management systems, as well as other security information like threat intelligence feeds. The goal is to verify what was prioritized from the network and endpoint data and to prescribe the correct response based on severity and priority. This stage requires human analysis to make decisions based on environmental context and business risk. Highly refined and verified alerts are passed on to Stage 3.
Stage 3: Coordination and response
In this stage, playbook automation receives the prioritized response. This includes endpoint and network alerts generated by network detection and response (NDR) and endpoint detection and response (EDR) tools based on their respective analytic capabilities. Automation and orchestration playbooks leverage the data provided from correlation and analytics. These playbooks coordinate an attack response across endpoints, networks, users, and application management systems. The responses are executed at machine speed to mitigate the attack spread and can include human decision points to throttle the level of automation to appropriate levels for the situation.
The high degree of integration and interoperability between these platforms enables organizations to implement detection and response in a very practical and manageable configuration. This minimizes the number of security tools and applications that are necessary to address the entire detect, decide and respond security cycle. This implementation also provides a higher level of maturity than most organizations currently achieve.
The approach does not just work in theory. It works in the real-world using NDR. We can look at metrics from existing organizations that deployed the Cognito Platform from Vectra to see the average workload reduction for detecting, triaging and prioritizing events by a Tier-1 security analyst.
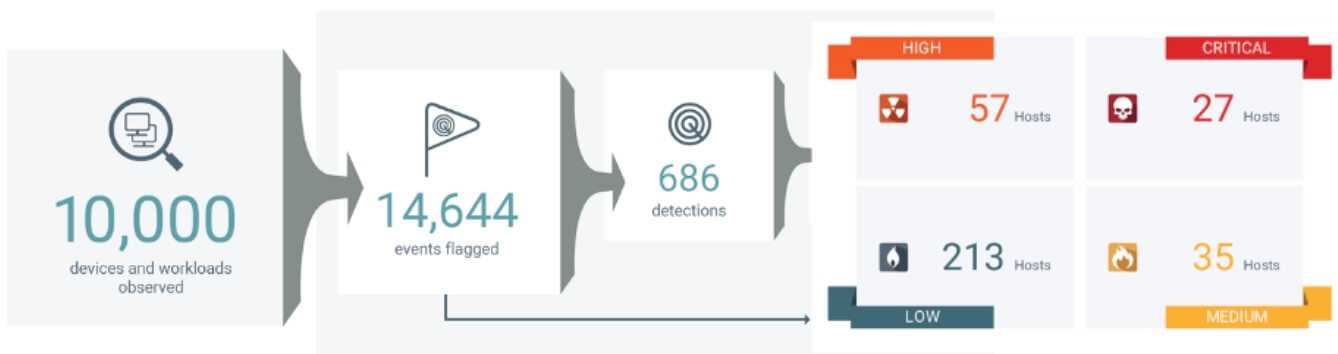
For every 10,000 devices and workloads monitored in one month, the average peak count of host severity flagged 27 critical and 57 high-risk detections. These devices and workloads present the greatest threat to an organization and require a security analyst’s immediate attention. Over a 30-day period, this works out to roughly one critical detection and two high-risk detections per day that require immediate attention. While other events may occur, few are of actual interest and should be escalated to senior analysts or business units for deeper investigation.
Behavior-based machine learning algorithms are incredibly useful in performing repetitive work at speeds faster than humans can possibly achieve around the clock and without errors. Machine learning delivers the deep insights and detailed context about in-progress cyberattacks, which enable security analysts to do the critical thinking to verify and to respond quickly to an incident. This is achieved by using a high-fidelity signal that filters out the noise that leads to false positives.
This in turn reduces the skills gaps and barriers of entry into security operations as a junior analyst while freeing up the time of highly skilled senior analysts to focus on threat hunting and acting as risk advisers to business units.
The takeaways
Here are three key points to remember.
Time is the most important metric for detecting and responding to attacks before damage occurs.
Stopping persistent and targeted attacks requires rapid detection and response.
Increased threat awareness and response agility are the outcomes of a mature incident response process.
Understanding risks in relation to the appropriate levels of threat awareness and response agility is vital.
Machine learning works best when applied to specific tasks.
It is well-suited to automating tedious, repetitive tasks while leaving the critical thinking and complex analysis to people.
If you need to improve your security operations and enhance your incident response capabilities, discover Vectra Advisory Services for a range of offerings tailored to your organization’s specific needs.